The Importance of Bounding Boxes in Data Annotation
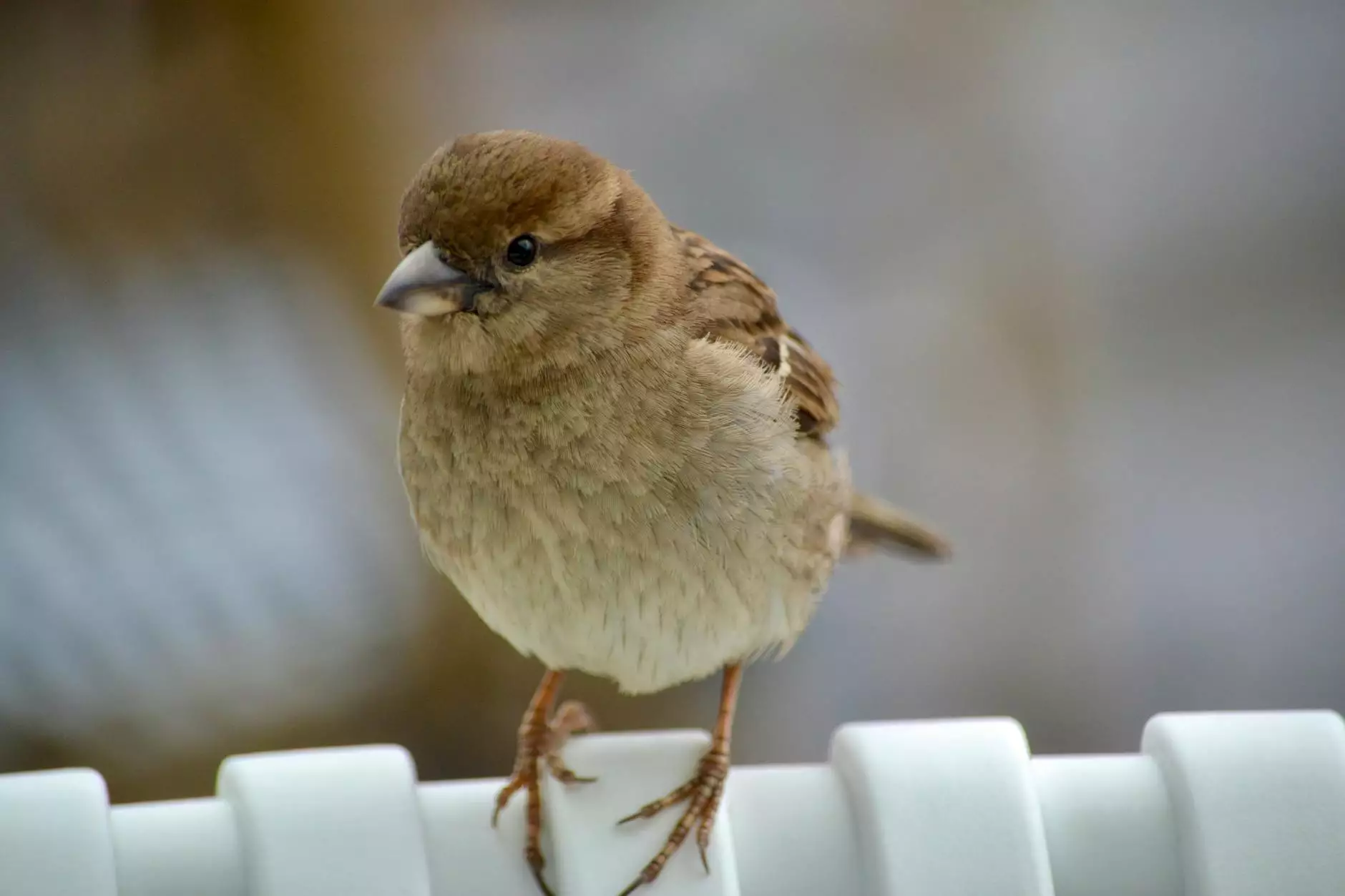
In the realm of data annotation, particularly in fields like computer vision and machine learning, bounding boxes serve as a crucial tool. They fundamentally enhance the accuracy and efficiency of various applications, ranging from autonomous vehicles to medical imaging analysis. This article aims to delve deeply into what bounding boxes are, their significance, and how businesses like Key Labs AI leverage these tools through their state-of-the-art data annotation platform and data annotation tools.
What Are Bounding Boxes?
Bounding boxes are rectangular frames used to define a portion of an image that contains an object of interest. The concept originates from the field of computer vision, where the primary objective is to enable machines to interpret and understand visual data as humans do. In practice, a bounding box is defined by two coordinates: the top-left corner (x, y) and the bottom-right corner (x', y'). This simple yet effective representation facilitates various tasks such as object detection, image segmentation, and tracking.
The Role of Bounding Boxes in Data Annotation
Data annotation entails labeling or tagging data to train machine learning models effectively. Bounding boxes play a pivotal role in this process for several reasons:
- Precision: The use of bounding boxes allows annotators to pinpoint the exact location of objects within an image, which is crucial for tasks like object detection.
- Scalability: Annotating images with bounding boxes can be done at scale, enabling the creation of large datasets that are vital for training complex models.
- Versatility: Bounding boxes can be adapted for various types of data, including images, videos, and 3D point clouds, making them an essential tool across multiple domains.
Applications of Bounding Boxes in Various Industries
The applications of bounding boxes are extensive and span across numerous industries. Here are some notable examples:
1. Autonomous Vehicles
In the automotive industry, bounding boxes are instrumental in developing autonomous driving systems. These systems rely heavily on computer vision to identify pedestrians, other vehicles, and obstacles on the road. By annotating training data with bounding boxes, machine learning models can learn to make real-time decisions based on visual input.
2. Healthcare and Medical Imaging
In healthcare, bounding boxes are deployed in analyzing medical images such as X-rays, MRIs, and CT scans. By accurately annotating regions of interest (like tumors or lesions) with bounding boxes, radiologists can enhance the diagnostic accuracy and provide better patient outcomes.
3. Retail and E-commerce
Retailers use bounding boxes to improve image search functionalities on e-commerce platforms. By annotating product images, businesses can implement more effective visual search algorithms, allowing customers to find products faster and enhancing the overall shopping experience.
Why Choose Key Labs AI for Data Annotation Services?
As the demand for precise and effective data annotation increases, Key Labs AI stands out as a leader in the industry. Here are several reasons why partnering with Key Labs AI can elevate your business projects:
- Advanced Technology: Key Labs AI utilizes the latest technology and tools to provide high-quality bounding box annotations that meet industry standards.
- Expert Team: Our experienced team of annotators ensures that every bounding box is meticulously placed, enhancing the quality of your datasets.
- Custom Solutions: We understand that each project is unique. That's why we offer customizable solutions tailored to meet specific project requirements, whether in computer vision, healthcare, or retail.
- Cost-Effective: We provide competitive pricing models, ensuring you receive top-notch annotation services without compromising your budget.
How Bounding Boxes Enhance Machine Learning Models
The integration of bounding boxes in training machine learning models significantly improves their performance. Here’s how:
1. Enhanced Accuracy
By accurately marking objects within images through bounding boxes, models can learn to differentiate between various classes with greater precision. This is particularly crucial in applications requiring high accuracy, such as medical diagnostics or security surveillance.
2. Improved Object Localization
Bounding boxes are essential for object localization tasks, where the goal is not only to identify what objects are present in an image but also to determine their precise locations. This leads to better performance in applications such as image segmentation and instance segmentation.
3. More Efficient Training
High-quality annotations speed up the training process of machine learning models. By providing clear and concise labels in the form of bounding boxes, models can converge more quickly and effectively.
Challenges in Using Bounding Boxes
While bounding boxes offer numerous advantages, there are also challenges associated with their use:
- Occlusion: Objects can obscure one another, complicating the task of annotators who must decide how to represent partially visible objects.
- Varied Aspect Ratios: Different object shapes may require multiple bounding box adjustments, leading to inconsistency in annotations.
- Labels' Accuracy: Mislabeling can lead to model inaccuracy; thus, consistent quality checks are necessary to maintain robust datasets.
Future Trends in Bounding Box Annotation
As technology evolves, so does the methodology surrounding bounding box annotations. Emerging trends include:
1. Automated Annotation Tools
The rise of artificial intelligence is paving the way for automated annotation tools that can generate bounding boxes with minimal human intervention. These tools utilize pre-trained models to aid in the annotation process, significantly reducing the time required.
2. 3D Bounding Boxes
With advances in consumer technology such as augmented reality (AR) and virtual reality (VR), there’s an increasing need for 3D bounding boxes, which encapsulate objects in three-dimensional space, offering a richer data representation for training models in these environments.
3. Integration with Blockchain
The integration of blockchain technology in data annotation systems provides a secure and transparent way to track annotations, ensuring data integrity and validation, which is especially critical in sectors like healthcare and finance.
Conclusion
Bounding boxes are a foundational element in the field of data annotation, providing clarity and precision that profoundly impacts the development of machine learning models. With businesses like Key Labs AI leading the charge in providing sophisticated annotation solutions, the future looks promising for both data scientists and industries relying on accurate computer vision capabilities.
As we continue to innovate and adapt our methods, it is imperative to understand and invest in high-quality data annotation practices. By embracing the essential role of bounding boxes in data annotation, your business can unlock new potentials and elevate its machine learning initiatives to unprecedented heights.